
ASHOK MUTTIN | April 20, 2022
If I had a dollar for every time
If I got a dollar for every time someone asked me what SupplyCopia (www.supplycopia.com) is doing in Machine Learning (ML) and Artificial Intelligence (AI), I’d be posting this article from my private island instead of New Jersey. But unfortunately, the issue has become the proverbial elephant in the room, and the answer surprises, maybe even shocks, most people.
There is much buzz and, to some extent, even hype around artificial intelligence (AI) and its power to revolutionize healthcare and the supply chain. Much of this buzz is created by providers of AI tools/technology, expensive consultants that have woven this into their PowerPoint presentations (and invoices), and so-called thought leaders who have taken it upon themselves to talk till they get blue in the face (something they’re very good at). Each seller’s most significant selling point is a combination of FOMO (fear of missing out) and the silver bullet, which solves all your problems (yes, including all your disconnected and dirty data).
Our industry is ripe with examples of experiments that have gone bad. Without naming names, let’s consider a few of them:
- A huge IDN is known for creating software products and then spinning them into separate companies. They developed an independent company, hired many industry experts, partnered with a leading AI technology provider, and marketed the solution as the next best thing since sliced bread. For 18-24 months, they decided to close the company, terminate their contract with their well-known AI partner, and bring all the people back into the parent company.
- A well-known cancer institute partnered with a leading AI technology provider to provide insights and treatment options to its physicians. This AI-powered technology was supposed to integrate multiple disparate data sources and provide insights and recommendations for treatment. After 12-18 months, this experiment was called off; the partnership was canceled.
- Leading IDNs created a coalition to pool their data (purchasing, patient demographics, reimbursements, outcomes). The objective was to create a “benchmark” that can be used by each organization and also sell this “tool” to other providers. Same story, same ending as the above two experiments. Difference? It took the coalition less than nine months to shut the initiative off!
Why did these well-funded, well-intentioned experiments fail? In each of the instances, they were unable to consider the quality and volume of the data and interoperability challenges. It’s one thing to win a Jeopardy competition, but it’s an entirely different story when working with disparate, non-contextual, non-standard, non-uniform sets of data.
In my role, I speak with many decision-makers and get to understand their vision. Most of them have their feet firmly on the ground and understand the challenges of implementing AI solutions. Sometimes, I meet a unique head of the supply chain who wants to create a “people-less supply chain.” In such situations, I do not know whether to cry or laugh.
While there is no argument about the potential of AI in the long term, we ought to ask ourselves a few fundamental questions.
- Do I have the necessary data foundation for leveraging AI?
- If the answer is NO, am I willing to invest in creating this foundation?
- Are we ready and willing to accept the consequences of unsupervised AI (Imagine a situation where an AI engine has changed the implant manufacturer without letting the physician know!)?
- How will we comply with all the regulatory and privacy requirements imposed by statutory organizations?
For some strange reason, there is this mad rush to eliminate people. How do you achieve it? Deploy AI solutions and automate the process! The answer cannot be farther away from the truth.
We at SupplyCopia have been experimenting with our machine learning and AI tools for four years. We started by building our core foundation, i.e., the “classifier” engine. Next, we realized that the answer lies in creating a Man+Machine combination where the machine does the heavy lifting to the extent it can and allows human intervention to override the machine-generated recommendation. We then leveraged significant machine learning to build a bill of material (BOM) based on utilization and pattern recognition. We then architected a recommendation engine that sits on top of a humongous amount of data.
After four years of solid work, our algorithms can provide relevant recommendations. We have reached levels of scalability where we feel confident to intake multiple large IDNs simultaneously. As far as unsupervised AI is concerned, we are at least 5-7 years away.
We encourage you to start your journey sooner than later. We are convinced that machine learning and AI have a role in transforming healthcare and the supply chain. However, be realistic about your unique challenges and the path you may have to build.
We are happy to share our journey, challenges, and success. We hope it will accelerate your journey. If you would like to discuss this, please feel free to reach me at [email protected]
More from SupplyCopia
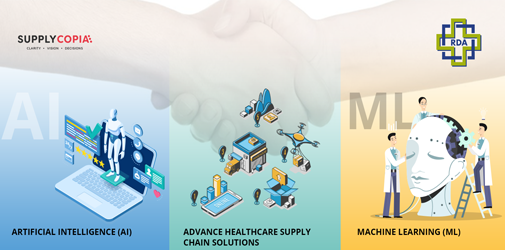
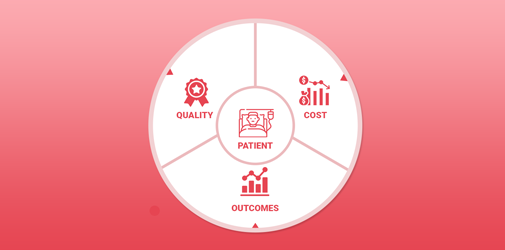